

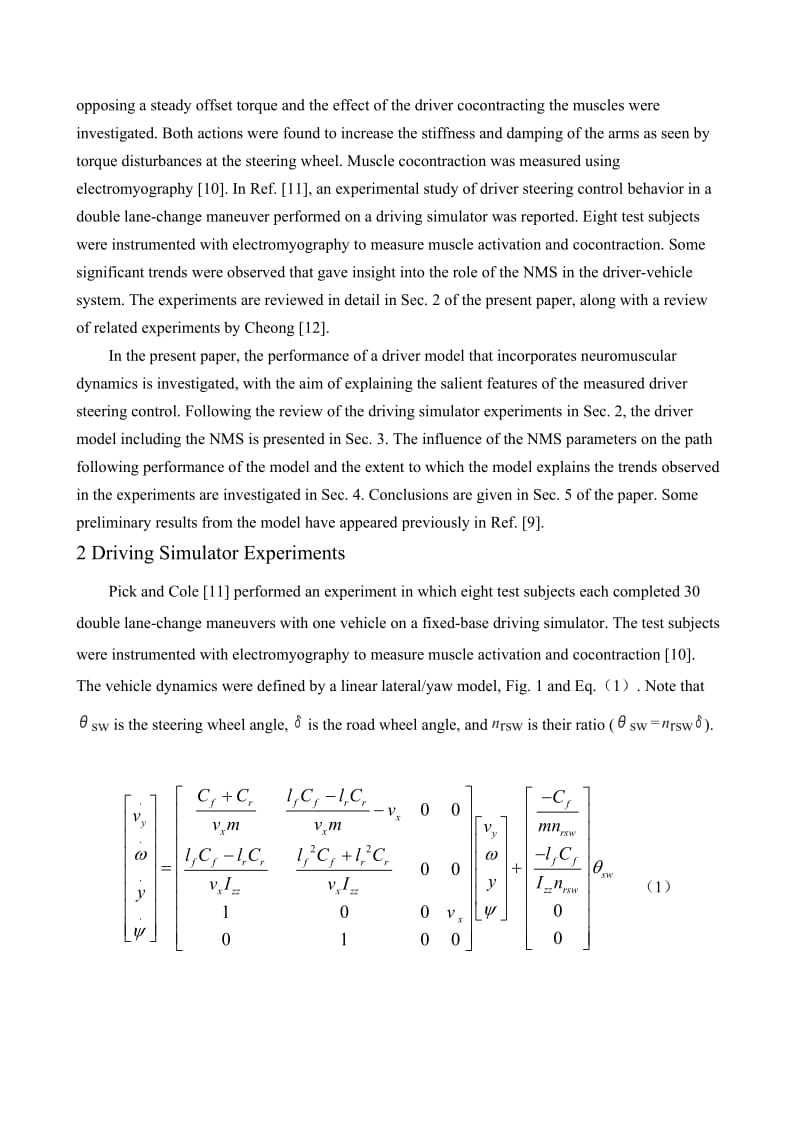


已閱讀5頁,還剩25頁未讀, 繼續(xù)免費(fèi)閱讀
版權(quán)說明:本文檔由用戶提供并上傳,收益歸屬內(nèi)容提供方,若內(nèi)容存在侵權(quán),請進(jìn)行舉報或認(rèn)領(lǐng)
文檔簡介
南京航空航天大學(xué)金城學(xué)院畢業(yè)設(shè)計(論文)外文文獻(xiàn)翻譯系 部機(jī)電工程系專 業(yè)車輛工程學(xué)生姓名俞斌峰學(xué)號2010012315指導(dǎo)教師毛建國職稱副教授2014年 2 月A Mathematical Model of Driver Steering Control Including Neuromuscular Dynamics Andrew J. Pick David J. ColeAbstractA mathematical driver model is introduced in order to explain the driver steering behavior observed during successive double lane-change maneuvers. The model consists of a linear quadratic regulator path-following controller coupled to a neuromuscular system (NMS). The NMS generates the steering wheel angle demanded by the path-following controller. The model demonstrates that reflex action and muscle cocontraction improve the steer angle control and thus increase the path-following accuracy. Muscle cocontraction does not have the destabilizing effect of reflex action, but there is an energy cost. A cost function is used to calculate optimum values of cocontraction that are similar to those observed in the experiments. The observed reduction in cocontraction with experience of the vehicle is explained by the driver learning to predict the steering torque feedback. The observed robustness of the path-following control to unexpected changes in steering torque feedback arises from the reflex action and cocontraction stiffness of the NMS. The findings contribute to the understanding of driver-vehicle dynamic interaction. Further work is planned to improve the model; the aim is to enable the optimum design of steering feedback early in the vehicle development process.Keywords: driver, steering, neuromuscular, muscle, reflex, torque, vehicle, feedback1 IntroductionThe introduction of driver assistance technologies like steer by wire is leading to a greater need to understand the way in which road vehicles operate in closed loop with the driver. Mathematical driver models have received increasing attention recently, see Refs. 14 for reviews of the literature. Developments include adaptive behavior, human control bandwidth limitation, and the improved use of path preview information. Despite this recent work, significant further progress is needed before sufficiently realistic driver models are available 4. In order to assess the full potential of new steering technologies such as electric power steering and steer by wire, it is necessary to understand the dynamic interaction between the vehicles steering system and the drivers neuromuscular system (NMS). The significant role that the NMS might play in driver steering control has previously been recognized by others 3,5,6.Pick and Cole 79 have described a method for identifying the dynamic properties of a drivers arms holding a steering wheel. A single degree-of-freedom linear mass-damper-spring model was found to fit well to the measured torque-angle transfer functions. The effect of the driver opposing a steady offset torque and the effect of the driver cocontracting the muscles were investigated. Both actions were found to increase the stiffness and damping of the arms as seen by torque disturbances at the steering wheel. Muscle cocontraction was measured using electromyography 10. In Ref. 11, an experimental study of driver steering control behavior in a double lane-change maneuver performed on a driving simulator was reported. Eight test subjects were instrumented with electromyography to measure muscle activation and cocontraction. Some significant trends were observed that gave insight into the role of the NMS in the driver-vehicle system. The experiments are reviewed in detail in Sec. 2 of the present paper, along with a review of related experiments by Cheong 12.In the present paper, the performance of a driver model that incorporates neuromuscular dynamics is investigated, with the aim of explaining the salient features of the measured driver steering control. Following the review of the driving simulator experiments in Sec. 2, the driver model including the NMS is presented in Sec. 3. The influence of the NMS parameters on the path following performance of the model and the extent to which the model explains the trends observed in the experiments are investigated in Sec. 4. Conclusions are given in Sec. 5 of the paper. Some preliminary results from the model have appeared previously in Ref. 9.2 Driving Simulator ExperimentsPick and Cole 11 performed an experiment in which eight test subjects each completed 30 double lane-change maneuvers with one vehicle on a fixed-base driving simulator. The test subjects were instrumented with electromyography to measure muscle activation and cocontraction 10. The vehicle dynamics were defined by a linear lateral/yaw model, Fig. 1 and Eq.(1). Note that sw is the steering wheel angle, is the road wheel angle, and nrsw is their ratio (sw = nrsw). (1)Fig. 1 Linear yaw/sideslip vehicle modelFor each driver, the steering torque feedback characteristic of the vehicle was changed after every ten maneuvers, but the vehicle response to steering wheel angle inputs was not changed (the ratio nrsw of steering wheel angle to road wheel angle was fixed at 16). The three different torque characteristics (subsequently denoted Car 1, Car 2, and Car 3) were achieved largely by increasing the torque feedback arising from the front axle slip angle. This means that the steering wheel torque required to perform the maneuver increased and the dynamics of the steering system became more oscillatory. The drivers were not told that changes to the torque characteristic would occur. Full details of the experiment and data analysis can be found in 11, including the method for dealing with intersubject variability in steering behavior.In general, drivers were found to steadily reduce path-following error over the first 20 lane-change maneuvers. Having completed 20 maneuvers, no further significant reduction in path error was observed 11.The changes in steering torque feedback after every ten maneuvers were not found to cause significant degradation of the drivers path-following control. This robust behavior suggested that drivers base their control on an understanding of the relationship between vehicle response and steering wheel angle rather than between vehicle response and steering wheel torque 11.Significant muscle cocontraction occurred when the drivers performed the lane-change maneuvers. Cocontraction was thought to be a mechanism consistent with increased control bandwidth and robustness. The test subject that displayed the greatest level of muscle cocontraction also generated the highest frequency control inputs to the vehicle 11.The level of cocontraction was observed to reduce with driver experience of each of the three torque characteristics. This result was interpreted as drivers cocontracting muscles initially to compensate for unfamiliar torque feedback and to ensure robust control, and as drivers learn the torque characteristic, less cocontraction becomes necessary 11.Cheong 12 subsequently performed some additional experiments using the same driving simulator in order to test Pick and Coles assertion that drivers base their control on an understanding of the relationship between vehicle response and steer angle rather than between vehicle response and steer torque. Initially, Cheong performed an experiment similar to Pick and Coles, in which the steering torque feedback characteristic was changed after every ten maneuvers, with the vehicle response to steering wheel angle remaining constant. In Cheongs experiments, the steering torque feedback characteristic was dominated by a simple stiffness term Kst that provided a self-centering effect (in contrast to Pick and Coles experiment where the torque was dominated by the front axle slip angle). The stiffness Kst was switched between values of 3 N m / rad, 10 N m / rad, and 20 N m / rad. Figure 2(a) shows the vehicle path during the first maneuver in each group of ten maneuvers. The figure shows that the vehicle path was little changed by the change in steering torque feedback. Cheong considered that these results were consistent with the results of Pick and Cole 11 and supported the idea that drivers are robust to changes in steering torque feedback.Cheong 12 then performed a similar experiment using a set of vehicles that required similar steering wheel torque input but significantly different amounts of steering wheel angle to perform the double lane-change maneuver. The ratio nrsw was switched after every ten maneuvers between values of 1, 16, and 50. The steering torque feedback was also changed, but in such a way that the steering wheel torque necessary to maintain a given road wheel angle remained constant. This was achieved by keeping constant the product of the steering wheel to road wheel angle ratio nrsw and the steering wheel stiffness Kst. The ratio nrsw = 1 required very small movements of the steering wheel to complete the lane- change maneuver, while the ratio nrsw = 50 required large movements (but not so large that the drivers had to change the position of their hands on the rim of the steering wheel).Figure 2(b) shows the vehicle path during the first maneuver after the steering ratio is changed. The figure shows that changes in ratio nrsw have a significant effect on the drivers ability to follow the road path, despite the steering torque needed to complete the maneuver being similar. Cheong considered that these data provided further evidence to support Pick and Coles assertion that drivers base their control on an understanding of the relationship between vehicle response and steer angle rather than between vehicle response and steer torque.Fig.2 Results of driving simulator measurements by Cheong 12 showing the average vehicle path during lane-change maneuvers performed by eight test subjects immediately after changes in the steering system. In (a), the steering stiffness Kst is switched between values of 3N m / rad,10N m / rad,and 20N m / rad, in the order given in thefigure; the steering angle ratio is fixed nrsw = 16. In (b), the steering ratio nrsw is switched between values of 1,16,and 50; the torque needed to maintain a given road wheel steer angle is fixed.Fig.3 Vehicle and previewed road path showing global position of vehicle and road at time kT where ypi is the road path lateral displacement and pi is the road path heading angle3 Driver ModelThe linear driver model presented in this paper is based on a path-following controller involving preview of the road path, feedback of vehicle states, and minimization of a cost function. The output of the controller is the desired steering wheel angle, which is transmitted to the steering wheel via the drivers NMS. The NMS model includes the effect of torque feedback from the steering wheel to the drivers arms. Details of the path-following control are given in Sec. 3.1 and details of the NMS model are given in Sec. 3.2.The controller is implemented in discrete time. The continuous time definition of the vehicle dynamics (1) can be converted to discrete time using the “c2d” function in MATLAB: x(k + 1) = Adx(k) + Bdu(k) (2)where k is the time step index.3.1 Path-Following Control. Sharp and Valtetsiotis 13 use linear quadratic regulator (LQR) control theory to derive a model of driver steering control that makes use of road path preview. Cole et al. 1 demonstrate that this LQR preview controller per- forms equivalently to a model predictive controller with long pre- diction and control horizons. The control model assumes that the driver previews the road at Np equally spaced points ahead of the vehicle, as shown in Fig. 3. The spacing between points is vxT, where vx is the forward vehicle speed and T is the time step for the discrete time model.Sharp and Valtetsiotis 13 show that by using a shift register operation, the road path preview can be incorporated with the vehicle state space equation (2): (3)whereyp(k) = yp0(k) yp1(k) yp2(k) yp(N1)(k) yp(N)(k)TA quadratic cost function J is used in calculating the optimal steering controller 13: (4)For path-following control, weights qy and q are placed on lateral position and heading angle errors, respectively. R2 is a scalar and is the weighting placed on the steering angle. In matrix form, the cost function is represented by (5)where z(k) = x(k) yp(k)T.R1 = CTQC,A LQR controller, Kp, can be calculated that minimizes the cost function J. The LQR controller can be calculated using the DLQR function of MATLAB, given R1, R2, and the composite vehicle and road path model (3). The optimal steering wheel angle 9 sw is then given by (6)where Kp = k1 k2 k3 k4 kp0 kp1 kpN.Kp consists of k1 k4, known as the state gains, which act on the vehicle states vy(k) 。(k) y(k) (k)T, and kp0 kpN, known as the preview gains, acting on the previewed path information.The vehicle model described by Eqs. (3) and (6) was used with parameter values given in Fig. 1 with discrete time step T= 0.02 s, steering gear ratio nrsw = 16, and vehicle speed vx= 38.9 m / s. The front and rear cornering stiffnesses were C f =2 X 88,310 N / rad and Cr =2 X 64,076 N / rad. The number of preview points was N = 200. In addition, a time delay of d= 0.16 s (corresponding to eight time steps) in applying the steering angle was included using another shift register, to represent the delay associated with human control 3. Gains for the LQR controller were evaluated with cost function weights qy = 0.25, q = 100, chosen to give good agreement between the measured 11 and simulated responses. Values of state gains and preview gains for the controllers without and with time delay are given in Ref. 2.The performance of the LQR controller with time delay, coupled directly to the vehicle (i.e., without the NMS, 9 sw = 9sw), can be compared with the mean path taken by all test subjects in all 30 lane-change maneuvers, Fig. 4. There is reasonable agreement between the model and measured data, but the measured mean path taken by all test subjects shows more overshoot and oscillation. This discrepancy will be seen to reduce when the NMS dynamics of the driver are included in the model3.2 Neuromuscular System Model. The path-following controller described in the previous section was augmented with a model of the NMS, as shown in Fig. 5. The steering wheel angle commanded by the path-following controller is transmitted to the vehicle via the NMS. Torque from the vehicles steering system is fed back into the NMS. It is assumed that the path-following controller has no knowledge of the NMS when calculating the optimal gains.The NMS model is developed from the work of Magdaleno and McRuer 14, who investigated the NMS dynamics associated with aircraft pilots controlling joysticks. The model in Fig. 5 represents the combined response of an agonist/antagonist muscle pair and therefore both positive and negative steering torques can be produced. Sections 3.2.1, 3.2.2, 3.2.3, and 3.2.4 describe each of the four blocks in the NMS of Fig. 5.Fig. 4 Mean path taken by test subjects through lane change and performance of LQR controller when linked directly to the vehicle. Error bars show 1 standard deviation from the mean path taken by test subjects.3.2.1 Arm and Steering Dynamics. In Ref. 7, it was shown that the dynamic response of the drivers arms to random torque disturbances applied at the steering wheel could be represented by a simple inertia connected to ground via a parallel spring and damper. The passive stiffness and damping of the arms in the relaxed state arise from the properties of the tendons, skin, and muscle. It is assumed that when the steering wheel is held at 0 deg rotation and the arms are holding the steering wheel at the “quarter to three” position, the muscles are at their resting (natural) length and relaxed. The values used in the model were taken from the measurements reported in Table 3 of Ref. 7, averaged over the eight test subjects: arm inertia Jdr = 0.064 kg m2, arm damping Bdr = 0.56 N ms / rad, and arm stiffness Kdr = 3.8 N m / rad, all referenced to the steering wheels axis of rotation. The steering sys- tem, including the steering wheel, column, rack and pinion, up- rights and wheels, and tires, can also be represented as a simple inertia Jst, damper Bst, and spring Kst system, where the spring represents the self-centering behavior. An additional torque feed- back term K is needed to represent the torque MT arising from the lateral forces and self-aligning moment generated by the slip angle f at the front axle, such that MT = K f . The values used in the simulation are given in Table 1 and correspond to the three torque feedback characteristics used in the experiments described in Sec. 2 and reported in Ref. 11.Fig. 5 Linear driver model with path-following control and NMS dynamics Table 1 Steering dynamic model parametersJst (kg m2)Bst (N ms/rad)Kst (N m/rad)K (N m/rad)Car 10.1721.562.291920Car 20.1720.982.293840Car 30.1720.4105760The arm and steering dynamics are coupled at the point where the driver grips the steering wheel. The equation of motion for the combined arm and steering dynamics is given by (7) and a trans- fer function is given by (8). (7) (8)3.2.2 Reflex Dynamics. The force producing fibers of the muscle are activated by alpha-motor neurons in the spine. The alpha-motor neurons can be signaled in two ways: directly from the brain and by feedback from spindles in the muscle. The spindles sense displacement and velocity of the muscle and feed back this information to the alpha-motor neurons to maintain muscle length at a value commanded by the brai
溫馨提示
- 1. 本站所有資源如無特殊說明,都需要本地電腦安裝OFFICE2007和PDF閱讀器。圖紙軟件為CAD,CAXA,PROE,UG,SolidWorks等.壓縮文件請下載最新的WinRAR軟件解壓。
- 2. 本站的文檔不包含任何第三方提供的附件圖紙等,如果需要附件,請聯(lián)系上傳者。文件的所有權(quán)益歸上傳用戶所有。
- 3. 本站RAR壓縮包中若帶圖紙,網(wǎng)頁內(nèi)容里面會有圖紙預(yù)覽,若沒有圖紙預(yù)覽就沒有圖紙。
- 4. 未經(jīng)權(quán)益所有人同意不得將文件中的內(nèi)容挪作商業(yè)或盈利用途。
- 5. 人人文庫網(wǎng)僅提供信息存儲空間,僅對用戶上傳內(nèi)容的表現(xiàn)方式做保護(hù)處理,對用戶上傳分享的文檔內(nèi)容本身不做任何修改或編輯,并不能對任何下載內(nèi)容負(fù)責(zé)。
- 6. 下載文件中如有侵權(quán)或不適當(dāng)內(nèi)容,請與我們聯(lián)系,我們立即糾正。
- 7. 本站不保證下載資源的準(zhǔn)確性、安全性和完整性, 同時也不承擔(dān)用戶因使用這些下載資源對自己和他人造成任何形式的傷害或損失。
最新文檔
- 二零二五電影制作保密合同范本6篇
- 二零二五版木材行業(yè)碳排放權(quán)交易合同范本8篇
- 2025年個人住宅房產(chǎn)抵押擔(dān)保合同范本
- 課題申報參考:內(nèi)感受干預(yù)促進(jìn)青少年情緒能力的神經(jīng)基礎(chǔ)
- 課題申報參考:民事訴訟法的基礎(chǔ)理論和基本制度研究
- 2025年度住宅小區(qū)停車位共有產(chǎn)權(quán)轉(zhuǎn)讓合同范本
- 2025年個人房產(chǎn)繼承權(quán)轉(zhuǎn)讓合同范本2篇
- 2025版農(nóng)機(jī)具租賃與智能灌溉系統(tǒng)合同4篇
- 二零二五版美容美發(fā)院加盟店會員管理與服務(wù)合同4篇
- 2025年度高端建筑用熱鍍鋅鋼管采購合同3篇
- DB43-T 3022-2024黃柏栽培技術(shù)規(guī)程
- 成人失禁相關(guān)性皮炎的預(yù)防與護(hù)理
- 九宮數(shù)獨(dú)200題(附答案全)
- 人員密集場所消防安全管理培訓(xùn)
- 《聚焦客戶創(chuàng)造價值》課件
- PTW-UNIDOS-E-放射劑量儀中文說明書
- JCT587-2012 玻璃纖維纏繞增強(qiáng)熱固性樹脂耐腐蝕立式貯罐
- 保險學(xué)(第五版)課件全套 魏華林 第0-18章 緒論、風(fēng)險與保險- 保險市場監(jiān)管、附章:社會保險
- 典范英語2b課文電子書
- 員工信息登記表(標(biāo)準(zhǔn)版)
- 春節(jié)工地停工復(fù)工計劃安排( 共10篇)
評論
0/150
提交評論